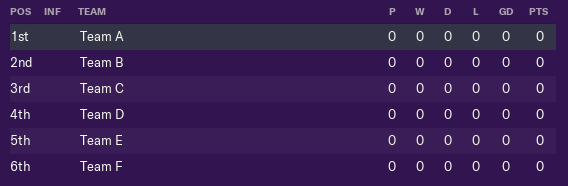
Professionalism in FM19: Welcome to the FM Stats Lab!
Statistics and myth-busting are the aim of the game in our FM Lab. We have set up a database and league to let us test what impact changing certain variables have on the actual game, to gather lots of data, and then test it statistically at the end. Specifically Professionalism in this first post. If like me you like seeing simulations and experiments, but also like me you want more hard figures then this is the experiment series for you. Professionalism in FM19.
There are plenty of posts, and videos out there with simulations and experiments. Some of them are fantastic windows into some of the elements, or variables, that might change the way we play but often for very practical reasons these will be only one season. Or they will be within the existing game world. That raises a few problems for nerds like me: sample size, variable control and actual statistical testing.
For example…
You want to know if teams with professional players are better than those without. You’ve changed the personality type for all the players in your chosen premiership team and then simulated a season to see where they finish.
Sample Size
- One season or so often isn’t enough in the way of data. That is a small number of matches, or data points, to draw conclusions from. You’ve got about 38 games plus any cup games. On a team level you’ve got approximately 40 points of data. Not terrible, but not great. The more data the better.
Variable Control
- You change your squad personality to see what impact that has and at the end of the season you can see your team as done better than most but not all of the other teams in the league. So you conclude professional squads do better. Success right? Not quite, you’ve changed your variable of interest but you’ve not controlled it for all the other teams and players in the game. Your team is interacting with lots of unknowns, there’s the influence of tactics, of the media, of reputation, of transfers, age, training, facilities, cup runs and morale… the list goes on and on. The good performance might be because of the personality change but it might also owe more to an unknown combination of all these other variables.
Statistics
- Ignoring the sample size and the control issue for a moment… Your team getting a good position in the league sounds interesting, but it’s not evidence yet that professionalism does have an impact. It could be a freak result, it could be by chance, or it could have an impact but in real terms it might have only been a tiny difference. This is where we need some statistical testing – some real nerdy statistics to not just describe the data but to let you know whether any differences and findings can be trusted.
Alternatively you could think about it another way: you want to know which antibiotics or medical treatment are best. You don’t just rely on one set of data, you control for as much as you can so you know any change is because of the pills, and you test to make sure you’ve got a reliable finding. We’re going to apply the same principle to FM.
The Experiment League
Our brave new lab world consists of an isolated experiment league, based in England. No promotion and relegation to mess things up, and no cups on the side to change the amount of games played. Remember it’s all about controlling our variables so we can see the impact of the few things we do change.
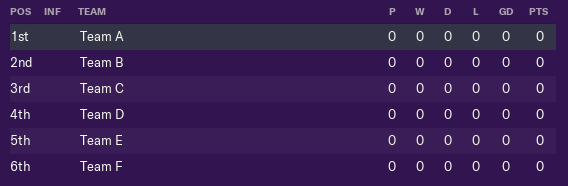
With six teams in the league, playing each other 8 times, we have a 40 game season.  It’s sorted on GD, goals for, and then at the end of the season results between teams, giving us a fairly standard set up.
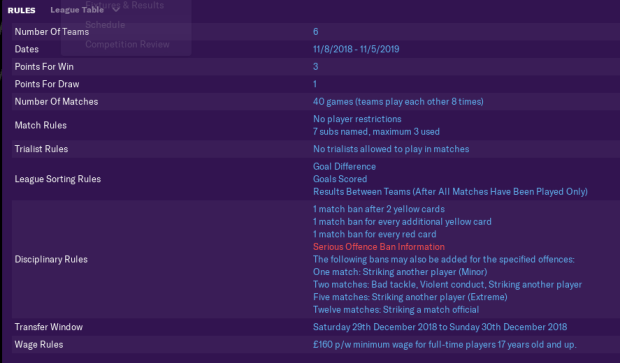
The Teams
Imaginatively named Team’s A to F are all based in Experiment City, each with their own equally imaginatively named 10k seater stadium. They are mediocre in every way (training, youth, data…), and are straight down the middle of every value including pitch condition and recovery.
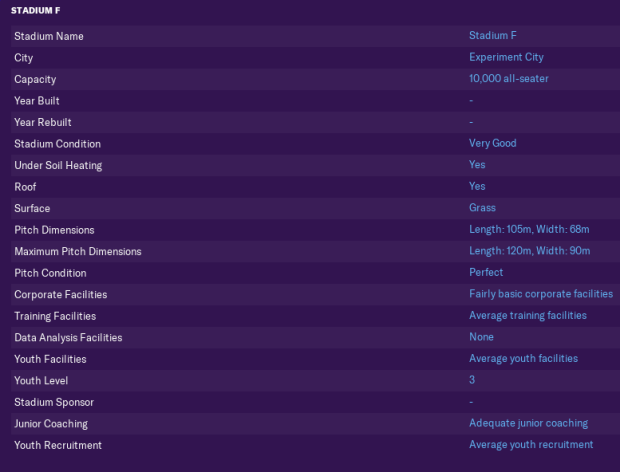
The Managers
Have you worked out the pattern?
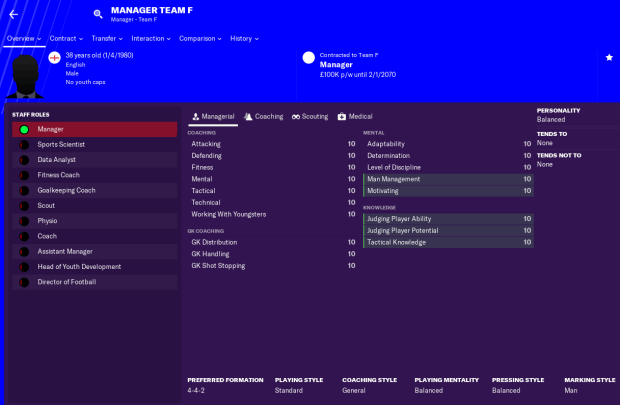
They are equally creative in that they all love the 4-4-2 and nothing but the 4-4-2. The fans and board love them, and they have a contract and wage that is costly enough to put off any thoughts of sacking during their first season in charge. However these are just backups – for any test where we want to try out a very particular tactic or squad selection, we can replace them with a player manager and then put them out on holiday for the season.
The Chairmen
Cut from the same cloth we have the chairmen of the clubs. Like club, manager and even the players (more on them in a second) the chairmen also embody middle of the road mediocrity. The only virtue they have is patience, with a solid 20 out of 20 to help ensure our managers stick around.
The Players
Each team has a squad of 24 players: Two keepers, four fullbacks, four centre backs, four wingers/wide midfielders, four central midfielders, one defensive midfielder, one attacking midfield and four strikers. Everything you could want for your robot/lab team.
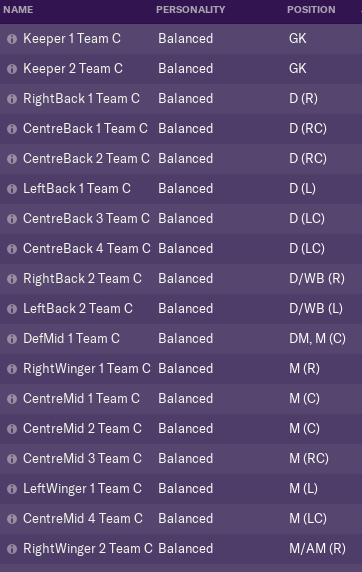
All the players have a PA of 100, and a CA of 100. Most physical and mental attributes are set to a safe yet boring 10-11 in the editor.
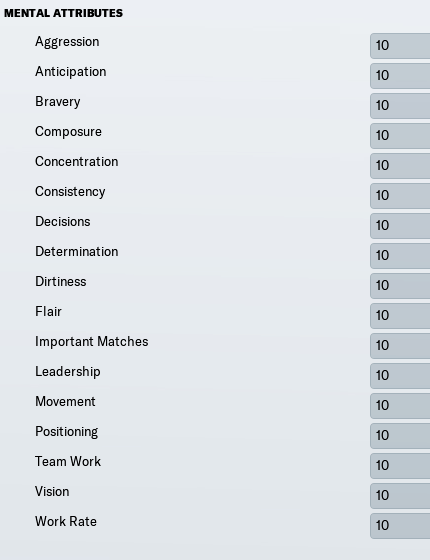
As a result position specific attributes are generally a 10-11, and everything else that doesn’t matter for the position is set as a 0 in the editor. This means every time the game is started a random value will be generated but it will be set by the available CA left. As most of the CA is in the already set values these random values tend to be pretty low.
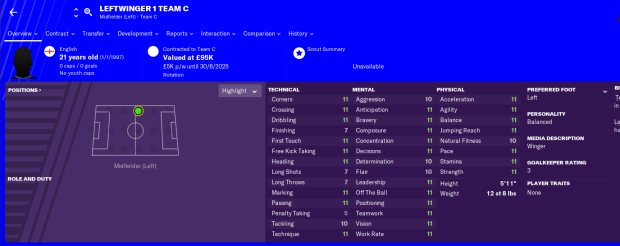
In our base database none of these players are world beaters. They are just blank, average, canvases for us to manipulate…for FM science.
What about: Transfers?

We’ve got a three pronged approach here to control transfers initially. All our teams have a transfer embargo, that can’t be appealed, and runs until 2030. If that wasn’t enough there’s only one day a year the transfer window is open anyway, New Years Eve. As a final line of defence if we really don’t want any transfer to take place we can add a human manager, but put them on holiday with the ‘reject transfers’ option selected. Nobody leaves.
What about: Europe?
As there are only six teams they would all qualify for Europe in some manner by simply existing. But for anyone worried that European qualification might be a rogue variable I have an extra file that wipes all European competition off the map. Welcome to our horrific footballing dystopia.
The Data
6 teams, playing 40 matches a season (each other 8 times), repeated for 10 seasons gives us a data set drawn from approximately 2400 matches (or 1200 unique matches). We can then analyse this at the team level (what impact does changing our variables have on teams overall) and the player level.
In the future if we want more we can add more teams, or more matches, or just more seasons. We have plenty of ways of upping the data if we need it.
Experiment 1: Impact of Professionalism
How does this Experiment League work in practice? Let’s try it with the example from above: What impact does professionalism have on team performance?
Firstly taking the base Experimental League I’ve made a simple change to our variable of interest (or Independent Variable for the true statistic nerds) of professionalism. All the players from Teams A and B have been given a high attribute score of 20. Those from C and D have kept our default value of 10, and those in Teams E and F have been given a low score of 1.
Then at the end of season 1 we will extract the data, and then restart and run the season again, until we have 10 sets of data. We can then look at some outcome variables (dependent variables for the nerds again): Position, Points, Goals For, Goals Against, GD, Red Cards, Yellow Cards, Wins, Draws and Losses.
Experiment 1: Professionalism Results

From table 1 it looks like the high professionalism group have generally out performed the others, grabbing more wins, fewer draws, and having a much healthier goal difference and points total. In fact it looks like there’s an almost 20 point difference between the high and low professionalism group.
The same picture can also be seen in the cards and fouls, with the lower professionalism seemingly corresponding with increased fouls and yellow cards.

Points aren’t everything though, as we know recently from the premiership title race sometimes all you get for 97 points is 2nd place. Lets look at table 2 then. The high professionalism sides grabbed 1st place 35% of the time, and finished in the top three 75% of the time. They never once, either Team A or Team B, finished in dead last.
Conversely the picture for the low professionalism side looks a little bleaker. Teams E and F never managed to finish 1st, and only made it into the top three 10% of the time.
But we don’t know if all of that reflects a real difference, a real impact of the professionalism attribute. It looks promising but we need to know more. Using special statistical software (SPSS – more on this another time) we can work out what differences, if any, are statistically significant. That is to say, which differences we can be relatively certain (above 95%) are real differences due to the change in professionalism rather than chance or other factors.
For the nerds in the back a series of ANOVA’s (with post hoc corrections) were run. I’ll explain more about that in a future post. We found a significant impact of professionalism on everything but the amount of games drawn. When we delve deeper we find that this difference reflects little difference between high (20) and medium (10) professionalism, but a big difference when compared to low (1). In other words 20 isn’t much better than 10, but medium or high are massively better than having low professionalism.
Why does Professionalism matter?
It is just an example but now I can be fairly certain that, all other things being equal, a professional squad is worth not just more than an unprofessional squad but that it is worth between 15-18 more points a season. Therefore I know how much value to assign to that when recruiting and building my squad. That difference, that edge, might be enough to swing a title race or a relegation fight. We know that professionalism is meant to be good, but now we know how good. In addition we also know that we get diminishing returns. It’s worth getting it to at least 10, but there’s not much value to be had increasing from 10 to 20 in this scenario.
Importantly we can do this with other attributes, with other combinations. We can look at manager influences or at club facilities. Any variable you can think of that can be tweaked in the editor we can play with in our Experiment League, our FM Lab, and see what impact it actually has.
In the future we are going to look at:
- Physical teams versus Technical teams
- Youth versus Experience
- The role of determination
- The impact of manager traits and ability
- If weather actually does anything?
- What combinations of mental attributes have the biggest impact on points won
And basically anything else anyone can think of. Comment below with suggestions
Make sure you follow us on our social media platforms and let us know if this article has helped you:
Other articles you may enjoy:
Decisions, Decisions, Decisions: FM Statistics Lab
Dictate the Game Podcast 11 | The FM Editor & DTG Cup
Dynamo Project: Introducing the Club DNA
Guide to the Wide Target Man and the Flo Pass Tactic