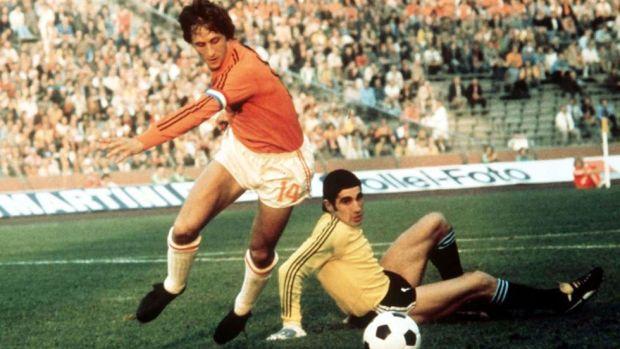
The importance of decision-making in FM19: FM Statistics Lab
Welcome back to the FM Statistics Lab. What’s the importance of decision-making in FM19?
In our first post we covered how we set up our lab environment to give is as much control as possible over our variables, and we also dipped our toes into the statistical water by investigating what sort of impact the professionalism attribute can have on overall team performance. If you’ve not had a chance to read it you can find it here – spoiler alert though, professionalism can have a huge impact. In this post we look in more depth at the Decisions attribute.
Our first post was a light introduction, and the statistics were kept on a leash, but now (for all you fellow nerds) we are going to delve a little bit deeper. If stats aren’t you thing that’s fine, you can still find out our headline results from each of our experiments and there’ll be a little glossary at the end of any statistical terms that get thrown around.
There have been some great suggestions in the comments so in this post we planned to cover two experiments:
- The Impact of Decision Making
- Physically, Mentally, or Technically good Strikers?
However we went down a stats rabbit hole with the investigation of Decisions. One that had originally been looked at by another blogger back in FM18 with their original version of an FM Lab. So the striker based experiments will have to wait until later…
Before we get going, I just want to cover something about how we are actually getting our results. I’m using SPSS. This stands for the Statistical Package for Social Sciences, and is basically Excel on steroids. Rather than just looking at averages, ranges, percentages (all stats we would call descriptive statistics) it can crunch the numbers through what are known as inferential tests or statistics. These are tests that let you know whether any difference found, or relationship, is significant or not. And by significant we mean it has a higher than chance liklihood of occuring, that it is probably a real difference or relationship rather than a freak occurance.
If you want to try using SPSS then you will need a license. Most Universities will have one so if you’re a student you’re covered. Alternatively there is an open source version called PSPP that has most of the same functions.
Experiment 2a: Decision Making
The decisions attribute is meant to cover the quality of the decisions made.
As the online FM manual points out it is:
The ability of a player to make a correct choice the majority of the time. This attribute is important in every position, and additionally works out how likely a player is to feel under pressure at any given moment, and to make the best choice accordingly.
There are others who have perhaps put it more poetically but the core principle remains the same.
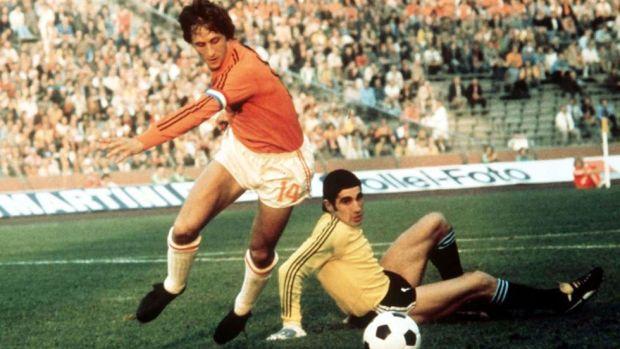
In other words a player with a good decisions attribute should be able to make better decisions, fewer mistakes, and generally have better outcomes. Better choices in theory should mean more key passes, tackles etc. that in turn influence the overall match outcome.
After our last post a few commenters from our opening stats lab post had mentioned past experiments that had suggested lower decision making was bizzarely related to better performance. This was a reference I think to a great post from FM18 by bluesoul that can be found here at Strikerless for their FM18 Lab (more on this later…)
Taking a similar approach to Experiment 1 in our opening post the editing Gods have blessed Teams A and B with 20 Decisions, Teams C and D with a middle of the road 10, and the 1 belongs to Teams E and F.
All teams are playing 442 and have the same infrastructure, reputation and the like, and all matches are run in full detail.
Results

As you can see from table 1 the evidence initially looks pretty damning. The descriptive statistics seem to suggest that the teams with 1 for their decisions attribute are massively and consistently out performing those with 10, who in turn are better than those with 20. There’s an approximately 40 point gap there!
This feels like madness. So much so that I paused after 7 trials. Teams A and B had finished in the bottom of the league table in each trial, and E and F had finished in the top 2 in all but one trial (where one of the 10 attribute teams snuck into 2nd).
Something didn’t feel right here but I ran the inferential statistics anway. I used an ANOVA, which stands for Analysis of Variance. This test lets you compare mean scores for 3 or more groups, and allows you to see if the difference in scores is statistically significant. That is to say if the change in the dependant variable can be attributed to a chance in the independant variable – or if the variance in our scores can be explained by the change in the decisions attribute. These tests give us a value, known as a significance value or a p value if this value is less than .05 ( p< .05) we can be fairly confident that we have found a real difference. Anything above .05 suggests no real difference.
Sure enough there was a statisitically significant difference. The difference in games won, drawn, lost, goals score, goals conceded and even goal difference was significantly different across the 3 groups with the low decisions teams doing better. P was less than .05 in every single case. Evening having 10 for decisions making was significanly better than having 20.
What does this mean?
Far from suggesting the decision attribute isn’t important or has no effect it seems to suggest that the opposite to what is expected is happening. The better the decision making the worse the performance. This really seems to fly in the face of the very definition of the attribute from SI but it did initially seem to confirm the findings of the original article by bluesoul, and their original FM Lab.
Completely confused I looked into the article by bluesoul, and the response on the SI forums. A key issue appeared, one that potentially blows apart the results.
Different attributes have different Current Ability weightings.
It even says so in the online FM guide:
The majority of Physical attributes, as well as Anticipation, Decisions and Positioning are the most heavily rated for any position, whilst each position carries appropriate weightings for attributes crucial to performing to a high standard in that area of the pitch.
That is to say that some attributes ‘cost’ more CA than others. Giving a boost to an attribute costs CA, and importantly reducing it free’s up CA. This excess CA then has an impact on the other attributes. Players have more ‘free’ CA so other attributes get boosted. Maybe only by a couple of CA points but by enough. According to one of the SI developers in the forum thread decisions is quite a heavily weighted attribute. This meant that our carefully controlled experiment had a spanner thrown in the works.
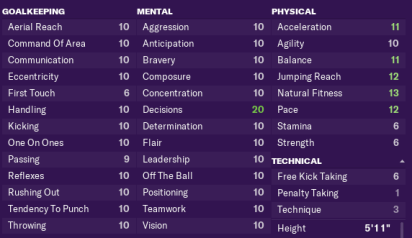
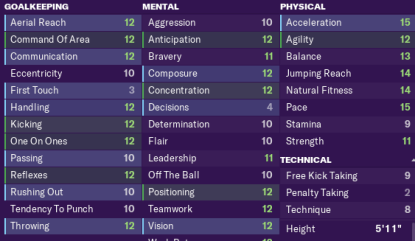
From first glance it was obvious. As you can see above a player from Team F had attributes 2-3 higher in places than a player from Team A. The extra CA to spare had been spent giving teams E and F a boost of about 2.5 attribute points on average, across the board, compared to teams A and B. No longer were we just testing the impact of the decisions attribute.
Looking in the editor made it even worse. The editor gives you a calculation of what a players CA should be based on the attributes already entered (the CA dependant attributes). On average players from teams E and F had 30 CA points to spare in comparision to teams A and B (who in some cases needed a boost of 8 CA points to account for the increased decisions attribute).
After all that experiment 1 was completely debunked purely because we hadn’t taken into account the editor. We’d done our best to control variables but it had gone awry. We could have saved a lot of time by reading through that thread. Let that be a lesson: reading first, editing later.
Experiment 2b: Decision Making
It’s not over though. We can put this question of the role of decisions to bed quite easily. Once you have changed your key attributes the editor tells you the recommended CA. All we had to do was then edit the CA and PA of each player to that suggested value. Decisions would remain the value we set, as would the other key attributes for the positions at 10, but adjusting the CA would mean no other attributes would get an unfair and unplanned boost.
For example, the suggested CA for our Keepers with 20 decisions was 108. But for the Keepers with 1 it was 60. A huge difference that explained our odd findings. These keepers had an extra 40 or so CA points to boost their other attributes in our original experiment. But by adjusting the CA, and PA to 108 and 60 respectively no other attributes would shift.
We made the changes and normality returned.
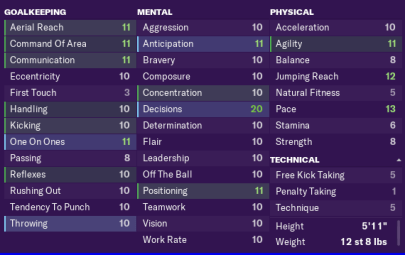
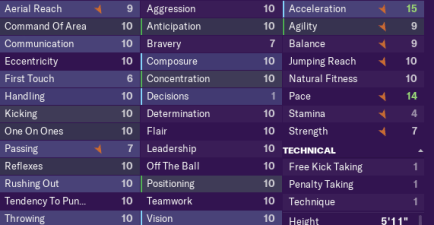
Results
We ran everything again, as before, and a drastically different picture emerged.

As you can see in table 2 the massive gap that existed before has now been massively reduced, to less than 3 points between high (20) and low (1). Now you may be thinking, that’s still a points difference. The low decisions teams are still doing better than the high. But remember these are just descriptive statistics, and 3 points is a small difference. The ANOVA will let us know whether this is a real and reliable difference.
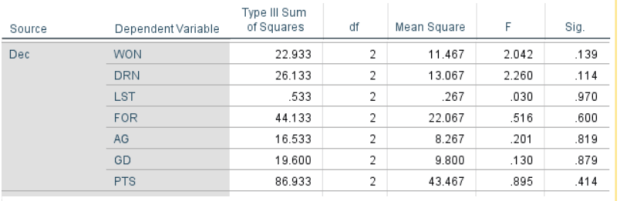
If our significance or p value is less than .05 we can conclude with some confidence that changing the decisions attribute has had an effect on our dependant variables like points, goals etc. But if we look at that far right column not a single value, from games won to points, is below .05. They are all above.
Therefore statistically what we have to conclude here is that changing the decisions attribute has had no effect on team performance. Vastly different from our initial findings. All other things being equal, the decisions attribute alone has no meaninful impact on team performance.
However what we don’t know though is how the decisions attribute interacts with other attributes. It’s hard to believe that it has no effect but it could be the case that it is a variable that interacts with others, or has it’s impact amplified under certain conditions.
Summary
It was a painful process but we learnt a few things here:
1. If someone suggests a replication of an experiment make sure you read around all the details so you don’t waste a day of editing and simulating!
2. It’s not enough to tell the editor the attributes if you want to fully control them. You have to adjust the CA and PA to stop the editor giving you a helping hand that actually ruins things.
3. Attributes aren’t equal. I don’t just mean in terms of how useful they are. Some ‘cost’ more, and sometimes this cost is also mediated by the position (defensive players had a different decision weighting than attackers).
4. Attributes don’t exist in isolation. It’s good to reduce variables and consider there impact of an attribute, one at a time, but ultimately some attributes are going to interact with others. We’ve not yet looked at that.
5. That the decision attribute really isn’t completely broken. The small differences suggested by the means differences might suggest theres a difference but statistically nothing is going on.
6. That my memory is terrible. Bluesoul not only ran a FM Lab like this well before me but I actually replied to one of the follow up posts of bluesoul and suggested looking into this in more depth with a form of analysis known as a mutiple or multi-linear regression! Bluesouls lab set up actually follows very similar principles to what I have here – which was completely unintentional on my part. My terrible memory aside there are key differences between the approaches. We have controlled the variables in slightly different ways, run the trials in a slightly different way, but most importantly for me are analysing these in very different ways. We are using inferential statistics here to determine whether any differences of effects found are ‘real’. Some similarities are also hard to avoid – if you want to take a more experiment based look at FM then you need a lab league or experimental league like the ones we both set up.
Next
For our next step we are going to move away from comparing the averages across groups, or changing single variables. We are going to look at more ‘natural’ sets and combinations of attributes and the impact they have on both team performance and on individual performance. In the next post I’ll be using the random ability ranges to fill in key attributes, running a lot of trials, and trying to determine:
- Which attributes predict key defensive performance statistics like key tackles, mistakes leading to goals, interceptions.
- Which attributes predict key attacking perfromances like minutes per goal, chances created, shots on target.
- How does the decisions attribute interact with other attributes (vision, technique etc.) to predict performance
This will be done with a form of analysis known as a multiple regression. It will allow us to see if we can predict a change in one variable (like goals for example) using a set of other known variables (like finishing, composure, anticipation). This lets us know if there is a relationship, and importantly what contributes most – essentially what are the better predictors.
If you have any suggestions let us know below.
Stats Terms
Descriptive Statistics
Stats such as means, medians, ranges etc. that help describe what your data looks like, and where differences and relationships MIGHT be. These statistics cannot be used to draw conclusions from. A difference in means between two groups does not mean that a real difference has been found.
Inferential Statistics
Tests that allow you to determine whether the differences in means etc. are statistically significant. That is to say whether they are real difference that reflect a real impact of changing your key variable rather than a chance result.
P Values/Significance
The value used to determine whether a significant finding is present. If the value is less than .05 you can be at least 95% certain a real statistical difference has been found. Above .05 and you treat this as if no difference has been found.
ANOVA
A type of inferential test that compares the means of 3 or more groups to determine if there is a significant difference between them.
Multi-Linear Regression
A form of analysis/test that allows us to determine whether certain groups of variables can significantly predict another.
SPSS
Software used for running statistical tests. Open source alternatives are available.
Make sure you follow us on our social media platforms and let us know if this article has helped you:
Other articles you may enjoy:
Professionalism: Welcome to the FM Statistic Lab!
Dictate the Game Podcast 11 | The FM Editor & DTG Cup
Dynamo Project: Introducing the Club DNA
Guide to the Wide Target Man and the Flo Pass Tactic
6 thoughts on “The importance of decision-making in FM19: FM Statistics Lab”
There’s a logical problem there. Raising decision making only is valuable if you can then execute on that decision. In other words, raising Decision on someone with 10 passing is not necessarily going to give you a better result. As the best pass “decision” is often a harder pass, hence prone to fail with an average passing.
To test this, I suggest doing the same experiment, but with one measurement difference:
You take a midfielder, only increase the decision stat for him (everyone else has 10s, him included in other stats), then measure the success of decision making by checking his average match performance.
You can even do this for 3 different players: one has low stats, one has medium stats, and the last one has high stats (in an average team).
Then of course run the season 10 times at least or so.
Otherwise, it’s like comparing how effective a dolphin is at climbing a tree. There’s no point testing decision making in places where it’s not relevant.
At least, your experiment showed that it’s not a straight up improvement if you don’t have the rest of the attributes to use it properly.
I’m biased of course because this is my favourite attribute. But, I’ve got a strong intuition this one is a game changer at high levels, especially combined with passing and creativity.